Introduction:
Data analysis is a powerful tool that can unlock valuable insights and drive informed decision-making. However, the success of any analysis hinges on one crucial factor: data quality. In the words of an unknown sage, “Remember, your analysis is only as good as the data you work with.” This statement resonates strongly with data analysts who understand the profound impact of data quality on the accuracy and reliability of their findings. In this blog post, we delve into the importance of data quality and why it should be a top priority for every data analyst.
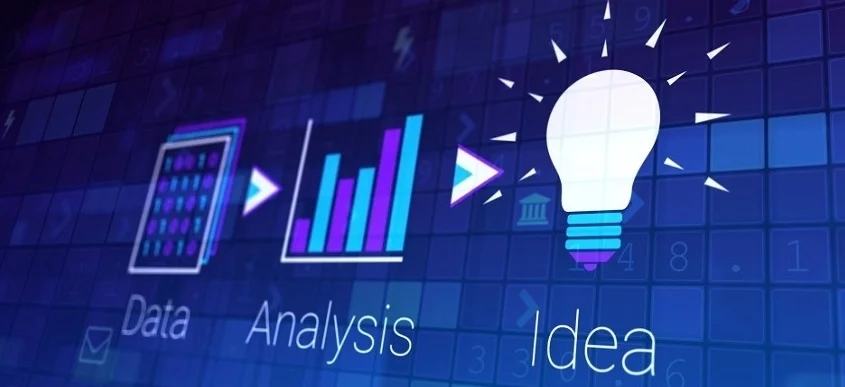
Why Data Quality Matters:
Data quality refers to the accuracy, completeness, consistency, and relevancy of data.
Here’s why it matters:
- Reliable Insights: High-quality data ensures the reliability of the insights derived from analysis. Flawed or incomplete data can lead to erroneous conclusions and misguided decisions. Data analysts must strive for data that is trustworthy and representative of the real world.
- Enhanced Decision-Making: Decision-makers rely on accurate and reliable information to make strategic choices. Data of poor quality can introduce biases, distortions, and incorrect assumptions, leading to suboptimal decisions. Data analysts play a crucial role in ensuring data quality to provide decision-makers with a solid foundation for their choices.
- Efficient Operations: Poor data quality can have cascading effects on business operations. It can result in wasted time, resources, and effort spent on analyzing and acting upon incorrect or irrelevant data. Data analysts can mitigate these risks by proactively addressing data quality issues and establishing robust data governance practices.
Ensuring Data Quality:
To prioritize data quality, data analysts should implement the following practices:
- Data Profiling: Conduct a thorough assessment of data quality by analyzing its structure, integrity, and consistency. Identify anomalies, errors, and outliers that may impact analysis.
- Data Cleansing: Employ data cleansing techniques to rectify inaccuracies, inconsistencies, and missing values. Remove duplicates, address outliers, and handle missing data appropriately to ensure a clean and reliable dataset.
- Validation and Verification: Validate the accuracy and completeness of data through various validation techniques. Cross-reference data against external sources, perform integrity checks, and verify data against known benchmarks.
- Documentation and Metadata: Maintain comprehensive documentation of data sources, transformations, and cleaning procedures. Capture metadata, such as data lineage, to provide transparency and enable reproducibility of analysis.
- Data Governance: Establish data governance frameworks and policies to ensure ongoing data quality. Implement data quality monitoring mechanisms, define data quality standards, and regularly assess and address data quality issues.
Conclusion:
Data quality is the bedrock of reliable data analysis. By prioritizing data quality, data analysts contribute to the integrity and trustworthiness of their analysis outcomes. As the custodians of data, it is their responsibility to ensure data accuracy, completeness, consistency, and relevancy.
By following best practices, employing data cleansing techniques, and implementing robust data governance, data analysts can empower decision-makers with accurate insights and drive meaningful impact through their work.
Let’s celebrate the importance of data quality during this Data Analyst Week and remember that our analysis is only as good as the data we work with!
#DataQualityMatters #DataAnalystWeek #DataAnalysis #DataQuality #ReliableInsights