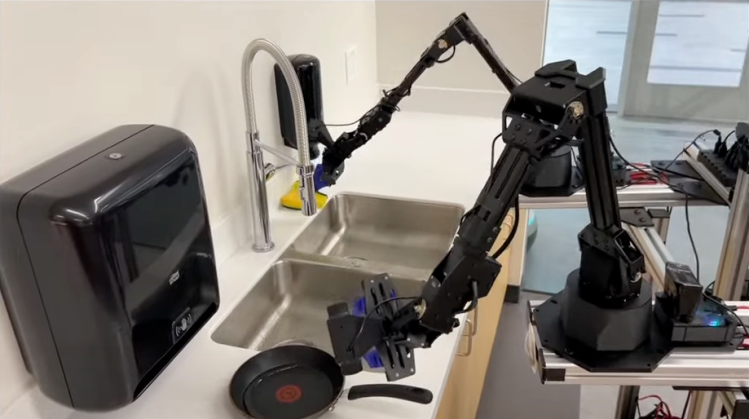
A new AI system developed by researchers at Stanford University makes impressive breakthroughs in training mobile robots that can perform complex tasks in different environments.
Called Mobile ALOHA (A Low-cost Open-source Hardware System for Bimanual Teleoperation) the system addresses the high costs and technical challenges of training mobile bimanual robots that require careful guidance from human operators.
It costs a fraction of off-the-shelf systems and can learn from as few as 50 human demonstrations.
This new system comes against the backdrop of an acceleration in robotics, enabled partly by the success of generative models.
Limits of current robotics systems
Most robotic manipulation tasks focus on table-top manipulation. This includes a recent wave of models that have been built based on transformers and diffusion models, architectures widely used in generative AI.
However, many of these models lack the mobility and dexterity necessary for generally useful tasks. Many tasks in everyday environments require coordinating mobility and dexterous manipulation capabilities.
Mobile ALOHA
The new system developed by Stanford researchers builds on top of ALOHA, a low-cost and whole-body teleoperation system for collecting bimanual mobile manipulation data.
A human operator demonstrates tasks by manipulating the robot arms through a teleoperated control. The system captures the demonstration data and uses it to train a control system through end-to-end imitation learning.
Mobile ALOHA extends the system by mounting it on a wheeled base. It is designed to provide a cost-effective solution for training robotic systems. The entire setup, which includes webcams and a laptop with a consumer-grade GPU, costs around $32,000, which is much cheaper than off-the-shelf bimanual robots, which can cost up to $200,000.

Mobile ALOHA configuration (source: arxiv)
Mobile ALOHA is designed to teleoperate all degrees of freedom simultaneously. The human operator is tethered to the system by the waist and drives it around the work environment while operating the arms with controllers. This enables the robot control system to simultaneously learn movement and other control commands. Once it gathers enough information, the model can then repeat the sequence of tasks autonomously.
Read more about it on Venturebeat